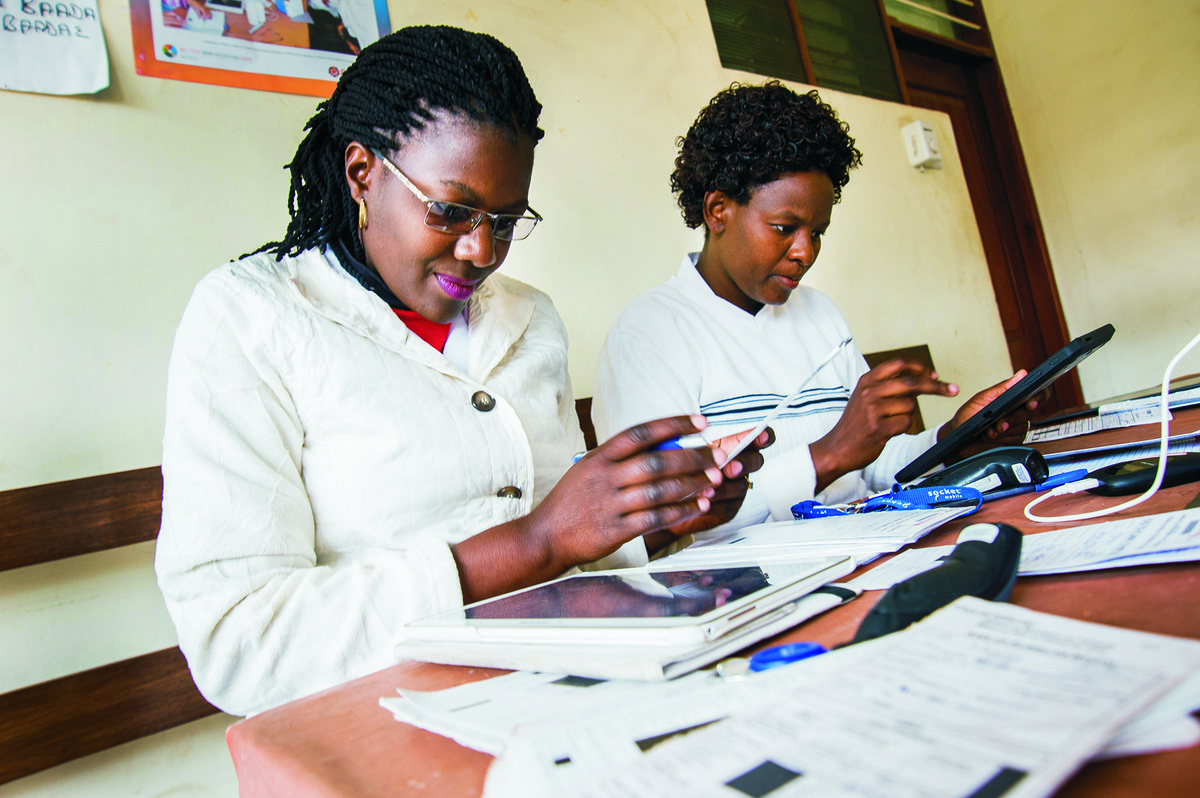
Large Language Models for Health Equity
Digital Square at PATH is working with a consortium of academic, government, technology, and implementing partners to conduct research, produce evidence and advance knowledge on the safe and effective use of large language model (LLM)-enabled tools in African health care settings. This work will ultimately support frontline health care workers to appropriately use LLMs to assist with clinical decisions, including patient diagnosis and treatment.
What is a large-language model (LLM)?
LLMs are applications of artificial intelligence that are trained using vast amount of data, designed to respond to queries and interact with the user like a human. Popular LLMs include ChatGPT and Google’s Gemini.
Can LLM-enabled tools support improved access to primary health care and delivery?
Many countries struggle to provide high-quality primary health care and achieve universal health coverage because of a shortage of trained clinicians. Consequently, citizens experience a variable standard of care depending on the level of training and experience of the health care workers in their community.
Appropriate and user-friendly tools are needed to provide on-demand, expert-level information, and support to frontline health care workers to standardize care and treat more patients effectively.
Artificial intelligence (AI)-based tools are increasingly being used to assist health care workers with diagnosis and treatment. These include LLM-enabled tools that can provide expert-level medical knowledge and advice in response to health care workers’ queries.
Because LLMs have the ability to be trained to think like a local medical expert, continuously learn, and provide responses to a wide range of queries, this technology is seen to have the potential to enhance clinical decision support systems and improve the quality of primary health care.
What we are doing
Digital Square at PATH is working with a consortium of academic, government, technology, and implementing partners to address three areas that currently make it challenging for low- and middle-income countries (LMICs) to effectively and safely utilize LLM-enabled tools in their health systems.
These challenges are:
- The absence of locally relevant datasets for training and evaluating LLMs.
- A critical lack of evidence on the appropriateness and effectiveness of LLM-enabled tools in health care settings in LMICs.
- Insufficient coordination and learning exchange among funders, implementers, government, the private sector, and local innovators.
Workstream 1
Developing local datasets for training and testing LLMs
AI-enabled tools are only as good as the data used to train them. Existing LLMs, much like other AI tools, are prone to bias as they are typically trained using data that is based on the health care challenges, protocols, and knowledge relevant to North America and Europe.
To ensure equitable access to LLM-enabled tools, our consortium of partners is collaborating in three countries—Kenya, Nigeria, and Rwanda—to create datasets (of medical questions and answers) using contextually relevant medical knowledge and practices.
Once the datasets are created, they will be made publicly available for others to train and finetune LLMs. The datasets will also be used to test the performance of existing LLMs, where an expert panel will compare LLM responses to responses provided by relevant medical experts.
Results will be published to allow others to learn from our approach and replicate it for benchmarking LLMs in other countries and contexts.
Workstream 2
Evaluating the accuracy and safety of LLM-enabled tools
A barrier to the use of LLM-enabled tools in primary health care settings in sub-Saharan Africa is the critical lack of evidence on the safety, appropriateness, and effectiveness of these tools.
Of the published randomized controlled trials focused on AI for health, only a small minority (two as of January 2024) were undertaken in Africa. Our consortium is undertaking a series of clinical trials to evaluate the performance of LLM-enabled clinical decision support systems for frontline health care workers in Kenya, Nigeria, and Rwanda, so that all stakeholders can more accurately understand the opportunities and challenges associated with this emerging technology.
Workstream 3
Community of practice
As part of this project, PATH has convened a community of practice that includes representatives from technology companies, donor organizations, implementing partners, and academia to facilitate a seamless exchange of cutting-edge knowledge and prevent duplication of efforts in this rapidly changing landscape.
The community of practice will engage through monthly virtual meetings to share challenges and learnings related to AI for health. Topics will include: data access and sharing; equity, user accessibility, and trust; and ethical and regulatory considerations.
News and Resources
-
Study protocol
A Multi-centre Randomized Controlled Trial in Kenya
-
Press Release
Clinical trial launches in Nairobi to generate evidence on the use of artificial intelligence in primary health care
-
Fact Sheet
Accelerating the Effective & Ethical Use of Large Language Models in Primary Health Care settings in sub-Saharan Africa
Our partners
This project is led by PATH, working in partnership with a consortium of partners including the University of Birmingham and Intron Health.
Partners supporting country-specific research and implementation include:
Rwanda
- Center for the Fourth Industrial Revolution Rwanda
- Digital Umuganda
- University of Global Health Equity
- Rwanda Biomedical Centre
Kenya
Nigeria
Meet the PATH team
-
Bilal Mateen, MBBS, MPH, PhD
Chief AI Officer
-
Mira Emmanuel-Fabula
Project Director
-
Jacqueline Deelstra
Senior Communications Officer
-
Avery Wilson
Project Manager
-
Celeste Gonda
Senior Project Administrator
Partner with us
Join us in accelerating research, learning, and ethical and appropriate LLMs for health.